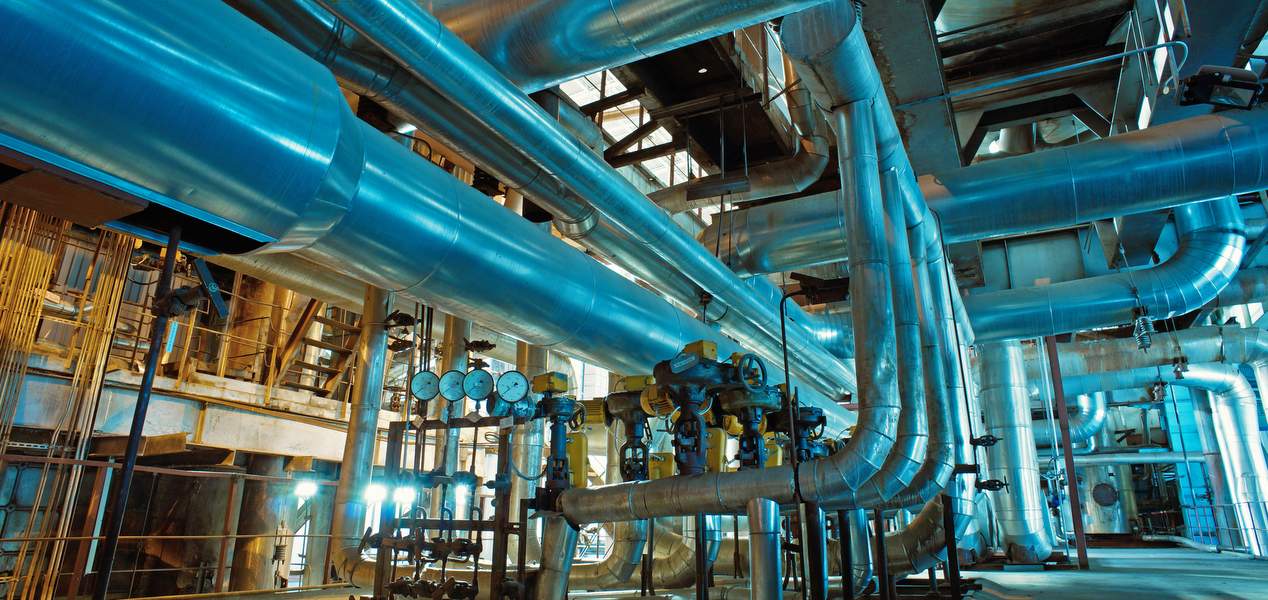
Use predictive modeling to control critical process parameters
The Big picture
A Fortune 100 fertilizer manufacturing company produces fertilizers that must meet quality criteria for key natural elements like potassium, nitrogen, and phosphorus to be within a defined range of specification. The quality of output is largely dependent on critical process parameters (CPPs) during the manufacturing process such as temperature, electric current, and flow volume. Using CPPs for predicting the output would help to keep the finished product grade within acceptable tolerance levels. Having a large number of CPPs, however, necessitates the need for a robust prediction model, which would balance out the trade-off between prediction accuracy and model complexity.
Transformative Solution
After thorough data understanding and brainstorming, the following four-step approach was taken:
- Harmonized the data to consolidate CPPs, and lab test the output into a single source of truth.
- Developed multiple random forest models for each output parameter and product grade.
- Validated the accuracy of the model on test data; then iterated and refine the model.
- Developed a final prediction summary and variable importance summary.
The Change
The results determined that for water soluble fertilizers, in 98.4% of instances the prediction accuracy was greater than 95%. For other nutrient products, in 99.9% of instances the prediction accuracy was greater than 95%. The client saw a multi-million-dollar savings through these tools in fine-tuning the manufacturing process, which statistically derived critical and controllable process parameters that drove the output grade.